Degenerative Spondylolisthesis: Web-Based Calculators and Outcomes
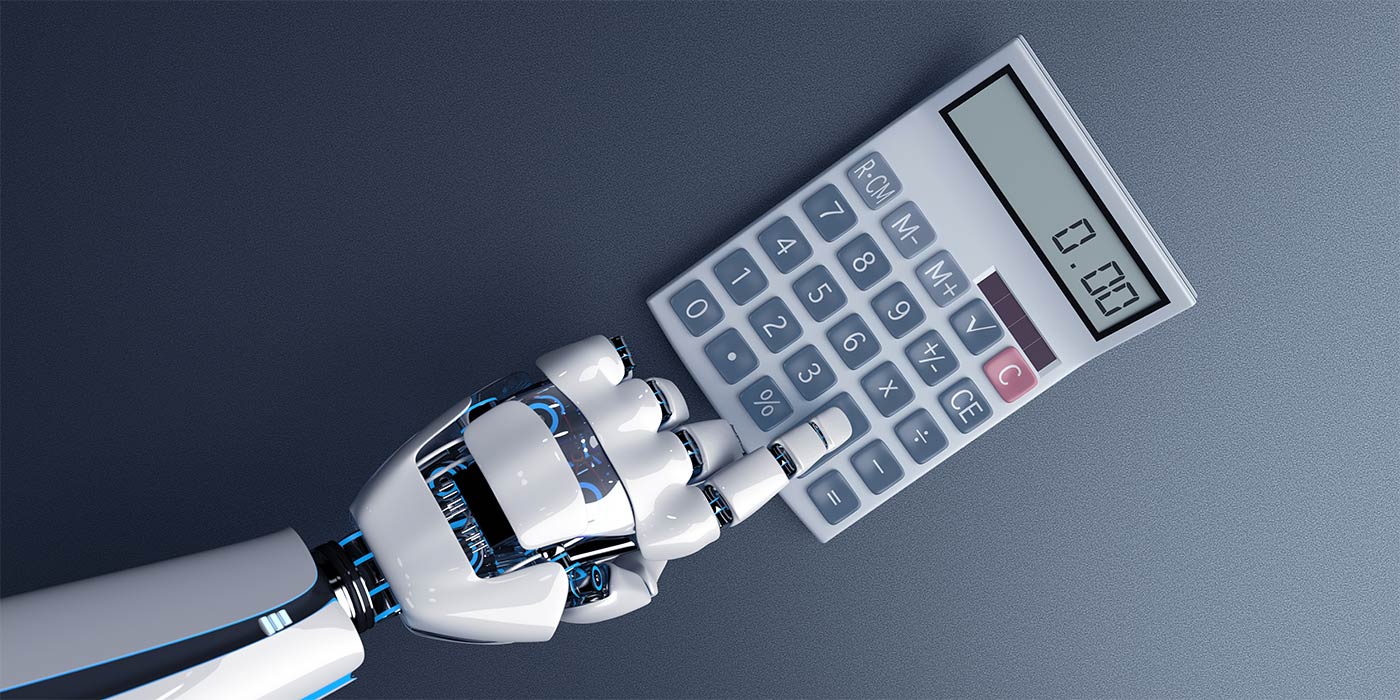
Written and edited by Nida Fatima, MD and Jakob Bower.
Degenerative spondylolisthesis (DS) is a segmental destabilization, which is the result of multifactorial degenerative changes in the spine. A number of factors has been associated with its development, including disc degeneration, facet joint orientation, gender, ligament hyperlaxity, and physical overactivity 1. The annual incidence of DS is approximately 266 million individuals worldwide 2, and it is estimated that the direct costs for treating these patients range from 80 to 100 billion dollars 3,4,5.
Despite the rising incidence and costs, there is considerable ambiguity regarding optimal management strategies for these patients. Symptomatic DS can be a result of spinal stenosis, typically causing limb symptoms, or mechanical back pain, impairing quality of life 6. Striking the right balance between different available treatment options is a difficult challenge. This review provides a holistic look at the etiology of DS and introduces some important tools that can be used to manage the condition and predict patient outcomes: web-based calculators powered by machine learning.
Factors involved in the pathogenesis of degenerative spondylolisthesis:
DS is historically considered a degenerative pathology with a ‘three-joint complex’—consisting of the intervertebral disc and two posterior zygapophyseal joints—that results in gradual destabilization of the spine 7. The incidence of DS increases with age in both sexes 8, however, females are more commonly affected by this pathology with an incidence of 12.7% 9
The presence of greater ligament laxity, hormonal effects, high pelvic incidence 10, L4 vertebral inclination, adjusted vertebral size, and facet orientation in the sagittal plane 9 were all independent predictors of the development of DS. Nonspecific degenerative lesions (joint hypertrophy, thickening of the ligamentum flavum, disc depression), facet joint sagittalization, and disc slippage predispose to canal narrowing and foraminal root compression among patients with DS 11,12.
Other complex factors, discussed in studies on muscular theory 13, disc theory 14, and hormonal theory, 15 are also thought to contribute to the pathophysiology of DS.
Treatment Options
Treatment options for DS include observation, physical therapy, orthotic management, and surgical intervention. Factors influencing decision-making for each treatment include age, concurrent medical comorbidities, magnitude of curvature, overall balance, and skeletal maturity.
Utilization of surgical data throughout history should enable a better understanding of current approaches and concepts of treating DS in a highly cost-effective manner. Presented below are three web-based calculators being used to gain further insights into clinical decision-making and determining DS patient outcomes.
Web-Based Calculators
Calculating Adverse Events following Surgery for DS: Fatima N et al 2020 16 recently developed a machine learning algorithm from large datasets based on multiple predictors for lumbar DS. This calculator provides predictive probability of the adverse events following surgery for patients with DS.
Calculating Discharge Disposition following Surgery for DS: In 2019, Karhade AV et al 17 developed a calculator using machine learning algorithms that can accurately predict discharge in patients undergoing elective surgery for DS.
Calculating Estimates of Personalized Treatment Outcomes: Moulton et al’s18 calculator, released in 2018, provides outcome prediction regarding the treatment of three back problems: slipped disc/sciatica, spinal stenosis, and slipped vertebrae.
In short, these web-based calculators can be incredibly useful tools for patient counselling and surgical risk assessment. The future of patient management lies in how we use innovations like augmented reality and artificial intelligence to support our surgical expertise. Combining all our data on clinical and radiographic factors will lay the foundation for better predictive outcomes and will help define the treatment plan for every patient suffering from DS. The future is all about AI!
References:
- Bernard F, Mazerand E, Gallet C, Troude L, Fuentes S. History of degenerative spondylolisthesis: From anatomical description to surgical management. Neurochirurgie. 2019;65(2-3):75-82. doi:10.1016/j.neuchi.2019.03.006
- Ravindra VM, Senglaub SS, Rattani A, et al. Degenerative Lumbar Spine Disease: Estimating Global Incidence and Worldwide Volume. Global Spine Journal. 2018;8(8):784-794. doi:10.1177/2192568218770769
- Dagenais S, Caro J, Haldeman S. A systematic review of low back pain cost of illness studies in the United States and internationally. The Spine Journal. 2008;8(1):8-20. doi:10.1016/j.spinee.2007.10.005
- Deyo RA. Trends, Major Medical Complications, and Charges Associated With Surgery for Lumbar Spinal Stenosis in Older Adults. JAMA. 2010;303(13):1259. doi:10.1001/jama.2010.338
- Weinstein JN, Lurie JD, Olson PR, Bronner KK, Fisher ES. United States’ Trends and Regional Variations in Lumbar Spine Surgery: 1992–2003: Spine. 2006;31(23):2707-2714. doi:10.1097/01.brs.0000248132.15231.fe
- Gille O, Challier V, Parent H, et al. Degenerative lumbar spondylolisthesis. Cohort of 670 patients, and proposal of a new classification. Orthopaedics & Traumatology: Surgery & Research. 2014;100(6):S311-S315. doi:10.1016/j.otsr.2014.07.006
- Kirkaldy-Willis WH, Wedge JH, Yong-Hing K, Reilly J. Pathology and Pathogenesis of Lumbar Spondylosis and Stenosis: Spine. 1978;3(4):319-328. doi:10.1097/00007632-197812000-00004
- Koreckij TD, Fischgrund JS. Degenerative Spondylolisthesis: Journal of Spinal Disorders and Techniques. 2015;28(7):236-241. doi:10.1097/BSD.0000000000000298
- Aono K, Kobayashi T, Jimbo S, Atsuta Y, Matsuno T. Radiographic Analysis of Newly Developed Degenerative Spondylolisthesis in a Mean Twelve-Year Prospective Study: Spine. 2010;35(8):887-891. doi:10.1097/BRS.0b013e3181cdd1aa
- Herkowitz HN, Rothman RH. Rothman-Simeone the Spine. Saunders/Elsevier; 2011.
- Vital J-M, Pedram M. Spondylolisthésis par lyse isthmique. EMC - Appareil locomoteur. 2006;1(1):1-20. doi:10.1016/S0246-0521(05)30353-6
- Grobler LJ, Robertson PA, Novotny JE, Ahern JW. Decompression for degenerative spondylolisthesis and spinal stenosis at L4-5. The effects on facet joint morphology. Spine. 1993;18(11):1475-1482.
- Ramsbacher J, Theallier-Janko A, Stoltenburg-Didinger G, Brock M. Ultrastructural Changes in Paravertebral Muscles Associated With Degenerative Spondylolisthesis: Spine. 2001;26(20):2180-2185. doi:10.1097/00007632-200110150-00003
- Inoue S, Watanabe T, Goto S, Takahashi K, Takata K, Sho E. Degenerative spondylolisthesis. Pathophysiology and results of anterior interbody fusion. Clin Orthop Relat Res. 1988;227:90-98.
- Sanderson PL, Fraser RD. THE INFLUENCE OF PREGNANCY ON THE DEVELOPMENT OF DEGENERATIVE SPONDYLOLISTHESIS. The Journal of Bone and Joint Surgery British volume. 1996;78-B(6):951-954. doi:10.1302/0301-620X.78B6.0780951
- Fatima N, Zheng H, Massaad E, Hadzipasic M, Shankar GM, Shin JH. Development and Validation of Machine Learning Algorithms for Predicting Adverse Events After Surgery for Lumbar Degenerative Spondylolisthesis. World Neurosurg. 2020 08; 140:627-641. PMID: 32344139.
- Karhade AV, Ogink P, Thio Q, Broekman M, Cha T, Gormley WB, Hershman S, Peul WC, Bono CM, Schwab JH. Development of machine learning algorithms for prediction of discharge disposition after elective inpatient surgery for lumbar degenerative disc disorders. Neurosurgical focus. 2018 Nov 1;45(5):E6.
- Moulton H, Tosteson TD, Zhao W, Pearson L, Mycek K, Scherer E, Weinstein JN, Pearson A, Abdu W, Schwarz S, Kelly M. Considering spine surgery: a web-based calculator for communicating estimates of personalized treatment outcomes. Spine. 2018 Dec 15;43(24):1731.